6 Agentic GenAI Guardrails You Absolutely Need (Or Face the Consequences)
Ignorance is NOT Bliss — Protect Your Business Today
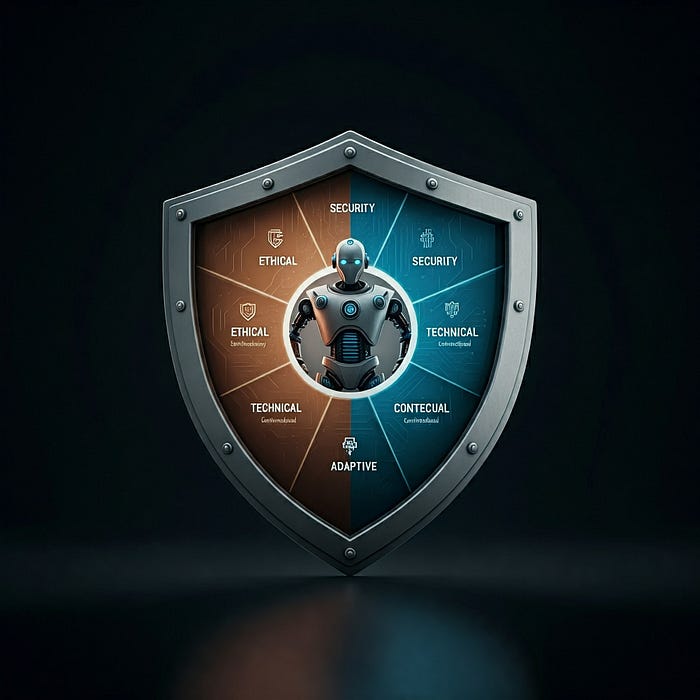
In the heart of Silicon Valley, amidst the towering tech giants and bustling innovation hubs, a revolution is brewing. A revolution led by the rise of Generative AI agents, poised to reshape our world in ways we’re only beginning to imagine. But with great power comes great responsibility, and the key to unlocking GenAI’s potential while safeguarding against its perils lies in the implementation of robust guardrails.
Think of GenAI agents as exceptionally talented but somewhat unpredictable teenagers. They can create stunning works of art, write captivating stories, and even generate complex code. But without proper guidance, they might inadvertently cross boundaries, produce harmful content, or make biased decisions. That’s where guardrails come in — the guiding principles, the ethical compass, and the security fences that ensure GenAI agents remain on the right track.
In this blog post, we’ll embark on a thrilling journey through the world of GenAI guardrails, exploring the six essential types that every organization needs to have in place. We’ll uncover the ethical dilemmas, security threats, and compliance challenges that GenAI agents can pose, and we’ll discover how guardrails can help us navigate these complex issues.
So, buckle up and get ready for an exhilarating ride through the world of GenAI guardrails. By the end of this post, you’ll not only understand the importance of these safeguards but also be equipped with the knowledge to protect your business and contribute to the responsible development of AI. Let’s dive in!
Ethical Guardrails — The Moral Compass of the GenAI World

Okay, let’s get this show on the road! It’s time to dive deep into the first type of GenAI guardrail: Ethical Guardrails .
Imagine an AI-powered hiring tool that automatically rejects applications from people with certain names because it was trained on biased data. Or a chatbot that starts spewing hate speech because it learned from toxic online forums. These are just a couple of examples of what can happen when AI systems lack ethical guidance.
Ethical guardrails are like the moral compass of an AI system. They help prevent the AI from producing outputs that are discriminatory, biased, or harmful. Think of them as the rules of the road that keep our AI driving in the right direction, ensuring it respects human values and societal norms.
Now, you might be thinking, “But how can an AI be unethical?” Well, AI systems learn from the data they’re trained on. If that data contains biases or reflects harmful stereotypes, the AI can inadvertently perpetuate those biases in its outputs.
The consequences of ignoring ethical considerations can be severe. Companies can face reputational damage, legal challenges, and even lose the trust of their customers. Remember the time a major tech company’s facial recognition software struggled to accurately identify people of color? That’s a prime example of why ethical guardrails are non-negotiable.
But here’s the kicker: defining ethical standards for AI is no walk in the park. What’s considered ethical in one context might not be in another. It’s like trying to hit a moving target while riding a rollercoaster! That’s why ongoing research, collaboration with ethicists, and a commitment to continuous improvement are crucial for building ethical AI systems.
So, what can you do to ensure your AI is ethically sound? Here are a few tips:
- Start with diverse and representative datasets. The more diverse your data, the less likely your AI is to develop biases.
- Involve ethicists in the AI development process. They can provide valuable insights and help you navigate ethical gray areas.
- Regularly evaluate your AI system for bias. Don’t just set it and forget it! Keep an eye on your AI’s outputs and make adjustments as needed.
Remember, building ethical AI is not just about avoiding negative consequences. It’s about creating AI systems that benefit society, promote fairness, and uphold human values. It’s about building AI that we can all be proud of.
Security Guardrails — The Guardians of the Agentic Galaxy

Alright, let’s shift gears and talk about Security Guardrails — the guardians of our AI systems, the sentinels that protect against those pesky cyber threats lurking in the digital shadows.
Imagine this: you’ve built an AI-powered chatbot to handle customer queries. But suddenly, a hacker injects malicious code into a user’s message, and boom — your chatbot starts spilling confidential customer data like a leaky faucet. That’s a security nightmare no one wants to experience.
Security guardrails are like the fortress walls and watchtowers of our AI systems. They protect against internal and external threats, ensuring that our AI doesn’t become a gateway for data breaches, misinformation campaigns, or other malicious activities.
Now, let’s talk about some of the villains in the AI security landscape:
- Prompt Injection Attacks: These are like sneaky spies that disguise malicious commands as innocent user inputs.
- Data Manipulation: Think of this as poisoning the well — attackers tamper with the AI’s training data or outputs, leading to disastrous consequences.
- Privacy Concerns: AI systems can sometimes be a bit too chatty, inadvertently revealing sensitive personal information.
- API Vulnerabilities: If our AI relies on external APIs, we need to make sure those APIs are locked down tight.
- Insider Threats: Sometimes, the enemy is within. Malicious insiders can exploit their access to wreak havoc on our AI systems.
- Jailbreaking: This is like breaking an AI out of its cage, allowing it to run wild and potentially cause harm.
But fear not! We have the tools to fight back:
- Input Validation: This is like a bouncer at a club, checking IDs and making sure only the right kind of data gets in.
- Data Encryption: We can scramble our data so that even if it falls into the wrong hands, it’s just gibberish.
- Access Controls: Only authorized personnel should have the keys to the AI kingdom.
- Regular Security Audits: Think of this as a routine checkup to make sure our AI’s defenses are still strong.
And here’s a bonus tip from yours truly: create strong, unique passwords for your AI systems. Don’t use your pet’s name or your birthday — those are rookie mistakes!
Investing in security guardrails is not just about preventing cyberattacks. It’s about protecting your reputation, maintaining customer trust, and ensuring the long-term success of your AI initiatives.
Compliance Guardrails — the rule books that keep our AI on the right side of the law

Alright, let’s get down to business and talk about Compliance Guardrails — the rule books that keep our AI on the right side of the law.
Imagine your AI-powered chatbot merrily chatting with customers, unknowingly revealing their credit card details or medical records. Yikes! That’s a compliance disaster waiting to happen.
Compliance guardrails are like the legal guardians of our AI systems, ensuring they adhere to all those rules and regulations that govern data privacy, intellectual property, and industry-specific guidelines. They’re like the seatbelts in our AI car, keeping us safe from legal collisions.
Now, let’s take a look at some of the compliance hurdles we need to watch out for:
- Data Privacy Violations: AI models are trained on massive datasets, and sometimes, sensitive personal information can sneak in. Compliance guardrails help us handle data responsibly and avoid those hefty fines that come with data breaches.
- Copyright Infringement: Our creative AI systems might inadvertently generate content that looks a little too similar to someone else’s work. Compliance guardrails help us steer clear of copyright infringement and protect intellectual property rights.
- Industry-Specific Regulations: Different industries have their own sets of rules for AI. Whether it’s healthcare (HIPAA), finance (KYC, AML), or marketing, compliance guardrails help us tailor our AI systems to meet those specific requirements.
So, how do we build AI systems that are compliant and law-abiding? Here are a few pointers:
- Define clear compliance policies: We need to know the rules of the game before we can play it. Organizations must establish policies that outline the specific compliance requirements for their AI applications.
- Implement robust data governance: We need to keep track of our data, where it comes from, how it’s stored, and who has access to it. Data governance is like the inventory system of our AI warehouse, ensuring everything is in its right place.
- Monitor and audit AI activities: We can’t just assume our AI is always playing by the rules. Continuous monitoring and auditing help us detect potential violations and keep our AI in check.
Investing in compliance guardrails is not just about avoiding legal trouble. It’s about building trust with your customers, protecting your brand reputation, and ensuring the long-term sustainability of your AI initiatives.
Technical Guardrails — the quality control inspectors of our AI workforce

Okay, let’s dive under the hood and explore the engine room of AI safety — Technical Guardrails .
Imagine an AI-powered news generator that starts producing articles about alien invasions and celebrity scandals, all fabricated from thin air. Or an AI code generator that spits out buggy code riddled with security vulnerabilities. That’s where technical guardrails come in to save the day.
Technical guardrails are like the quality control inspectors of our AI workforce, ensuring they produce accurate, reliable, and high-performing outputs. They’re like the brakes on our AI car, preventing it from spinning out of control.
Now, let’s take a closer look at some of the technical glitches we need to watch out for:
- AI Hallucinations: Sometimes, AI models can get a little too creative, generating false or misleading information that seems plausible but is completely made up. Technical guardrails help us fact-check those AI outputs and keep them grounded in reality.
- Inconsistent Output Formats: Imagine an AI code generator that produces code that can’t even compile! Technical guardrails help us enforce consistent output formats and structures, ensuring our AI produces usable and compatible results.
- Model Drift: AI models can change over time as they learn from new data. This can lead to performance degradation or unexpected behavior. Technical guardrails help us monitor for model drift and keep our AI on track.
So, how do we build AI systems that are technically sound and reliable? Here are a few tricks of the trade:
- Choose the right AI models: Not all AI models are created equal. Selecting the right model for the job is crucial for ensuring accuracy and reliability.
- Train on high-quality data: Garbage in, garbage out, as they say. Training our AI models on high-quality, unbiased datasets is essential for preventing errors and inconsistencies.
- Monitor AI performance: We can’t just assume our AI is always performing at its best. Continuous monitoring helps us detect technical issues and ensure our AI is running smoothly.
Investing in technical guardrails is not just about preventing technical glitches. It’s about building trust with your users, ensuring the accuracy and reliability of AI outputs, and maximizing the return on your AI investments.
Contextual Guardrails — the social etiquette guides of our AI workforce

Okay, let’s step into the realm of social graces and conversational etiquette with Contextual Guardrails .
Imagine asking your AI assistant about the weather and receiving a lecture on the political climate instead. Or worse, imagine an AI tutor teaching your little brother inappropriate vocabulary words! That’s where contextual guardrails come in to ensure our AI systems understand and respond appropriately to different contexts and situations.
Contextual guardrails are like the social etiquette guides of our AI workforce, teaching them the proper way to interact with humans in various settings. They’re like the navigation system in our AI car, guiding it along the appropriate conversational routes and preventing it from veering off course.
Now, let’s explore some common contextual faux pas that we need to prevent:
- Irrelevant Responses: AI models can sometimes lose track of the conversation’s focus and provide irrelevant or off-topic responses. Contextual guardrails help them stay on track and maintain the conversation’s coherence.
- Inappropriate Content: AI systems interacting with children or in professional settings should avoid sensitive or offensive language. Contextual guardrails help them maintain appropriate behavior and adhere to social norms.
- Misleading Information: AI systems providing information on sensitive topics like healthcare or finance should stick to verified sources and avoid offering unproven remedies or financial advice. Contextual guardrails help them maintain accuracy and credibility.
So, how do we train our AI systems to be contextually aware and socially adept? Here are a few tips:
- Topic Modeling: By analyzing and categorizing different topics, we can help AI systems identify when the conversation is veering off course.
- Keyword Filtering: Implementing lists of relevant keywords and phrases can help AI systems identify and flag responses that contain unrelated terms.
- Contextual Embeddings: Representing words and sentences as vectors that capture their meaning allows AI systems to measure the semantic similarity between user input and AI output, ensuring the response remains contextually relevant.
Investing in contextual guardrails is not just about preventing awkward conversations. It’s about enhancing user experience, improving efficiency in task-oriented applications, and maintaining trust and reputation by ensuring AI systems provide relevant and appropriate responses.
Adaptive Guardrails — the self-learning sentinels of our AI workforce

Okay, now it’s time to step into the future of AI safety with Adaptive Guardrails — the self-learning sentinels of our AI workforce.
Imagine a world where our AI systems can automatically adapt to new threats, evolving ethical standards, and changing user preferences. That’s the promise of adaptive guardrails — the dynamic, context-aware safety mechanisms that go beyond static rules and evolve alongside our AI systems.
Adaptive guardrails are like the self-learning sentinels of our AI workforce, constantly monitoring, analyzing, and adapting to new challenges and opportunities. They’re like the self-driving system in our AI car, navigating the ever-changing landscape of the digital world with autonomy and intelligence.
Let’s explore some examples of how adaptive guardrails can revolutionize AI safety:
- Dynamically Adjusting Content Filters: As AI models encounter new forms of harmful or inappropriate content, adaptive guardrails can update their filtering mechanisms to block these emerging threats, ensuring ongoing protection against evolving risks.
- Adapting to User Feedback: AI systems can learn from user feedback and preferences, dynamically adjusting their behavior to better align with user expectations. Adaptive guardrails ensure that this feedback is incorporated responsibly, preventing AI systems from being manipulated or exploited.
- Evolving Ethical Standards: As societal norms and ethical considerations change, adaptive guardrails can be updated to reflect these changes, ensuring AI systems remain aligned with current ethical principles.
- Detecting Novel Attacks: Adaptive guardrails can use machine learning to identify patterns indicative of new attack vectors or malicious intent, proactively detecting and mitigating threats that static rules might miss.
- Responding to Changes in Data: As AI models are exposed to new data sources or as the distribution of training data shifts over time, adaptive guardrails can adjust their monitoring and validation processes to ensure the model’s outputs remain reliable and relevant.
Use cases for adaptive guardrails span a wide range of applications:
- Personalized Learning Platforms: AI tutors can dynamically adjust their teaching strategies and content recommendations based on individual student progress and feedback, while adaptive guardrails ensure the AI tutor provides appropriate and relevant educational material.
- Evolving Chatbots: Customer service chatbots can learn from past interactions and user feedback to improve their responses, while adaptive guardrails ensure the chatbot stays on topic and avoids offensive language.
- Content Moderation on Social Media: AI systems can detect and flag harmful content, while adaptive guardrails dynamically adjust content filters based on emerging trends in online abuse and evolving community standards.
Building adaptive guardrails requires careful consideration of several factors:
- Monitoring and Feedback Mechanisms: Establish systems for continuous monitoring of AI behavior, collecting user feedback, and incorporating insights from security research.
- Machine Learning Integration: Leverage machine learning algorithms to analyze patterns, identify anomalies, and adapt guardrail parameters based on evolving threats and changing contexts.
- Explainability and Transparency: Design adaptive guardrails with explainability in mind, allowing human operators to understand how the guardrails are making decisions.
- Human Oversight: Retain human oversight capabilities to review guardrail decisions and intervene in high-risk situations.
Investing in adaptive guardrails is an investment in the future of AI safety. By providing a dynamic and context-aware approach to safety, these guardrails empower organizations to harness the power of AI while mitigating evolving risks, adapting to change, and fostering trust.
Conclusion — Towards Safer GenAI Agentic Workflows
We’ve journeyed through the ethical dilemmas, security threats, compliance challenges, technical glitches, and contextual faux pas that can arise in the world of generative AI. We’ve explored the six essential guardrails that can help us navigate these complexities and ensure our AI systems remain safe, responsible, and beneficial to society.
Remember, building AI is not just about creating intelligent machines. It’s about creating machines that are ethical, secure, compliant, reliable, contextually aware, and adaptable. It’s about creating AI that we can trust, AI that enhances our lives, and AI that contributes to a better future for all.
So, as you embark on your own AI adventures, remember the lessons we’ve learned together. Keep those guardrails strong, stay vigilant, and never stop learning. The world of AI is constantly evolving, and it’s up to all of us to ensure its responsible development.
Now, I’d love to hear from you! What are your thoughts on GenAI guardrails? Have you encountered any examples of AI gone wrong? How do you think we can best ensure the safe and ethical development of AI? Share your thoughts and comments below — let’s keep the conversation going!
Disclaimers and Disclosures
This article combines the theoretical insights of leading researchers with practical examples, and offers my opinionated exploration of AI’s ethical dilemmas, and may not represent the views or claims of my present or past organizations and their products or my other associations.
Use of AI Assistance: In preparation for this articles, AI assistance has could have been used for generating/ refining the images, and for styling/ linguistic enhancements of parts of content.

This story is published on Generative AI. Connect with us on LinkedIn and follow Zeniteq to stay in the loop with the latest AI stories.
Subscribe to our newsletter and YouTube channel to stay updated with the latest news and updates on generative AI. Let’s shape the future of AI together!
